Iterating: shorter, more frequent outlooks
Conditions dry out across Northeast & Mid-Atlantic on Thursday, but not before another day of thunderstorms on Wednesday
We’re gonna try something different. It’s the benefit (and imperative) of a startup: we get to readily test what works best for our readers/users. (To that end, we’d love to hear from you!)
Most materially, we’re going to improve our weekday coverage. Rather than a deep dive into two or three days per month (typically Fridays or Sundays), we’ll provide a [shallower] two-day lookahead three times per week. Accordingly, this Tuesday morning email (surveying travel on Wednesday and Thursday) should become regular; as will emails on Thursday and Saturday mornings that preview the next day and day-after-next. That leaves Tuesday travel uncovered, though it’s been the most lightly traveled and least disrupted weekday1 during the last year. If you’re anxious about a trip on Tuesday, reach out—we’re happy to take a look.
We’ve refrained from a fourth weekly email because we’re admittedly a bit concerned about inbox clutter. So if you’re about to hit the unsubscribe button for that reason, please let us know. We can move these outlooks to a new, separately-subscribed-to, section of our Substack should feedback point us in that direction. Posts like our seasonal outlooks, musings on EWR or pilot population prognostications are unaffected by this mini-pivot. And we may still author the occasional one-day preview modeled after the original outlooks or write about those airports outside the scope of our new automation…
… which leads us to our narrowed scope. To enable more frequent posts, we’ve hacked together a bit of automation that ingests the National Blend of Models (NBM) forecast, finds past hours with similar weather conditions to those forecast, then evaluates capacities in those similar past hours against scheduled2 demand for that future hour. When more flights wish to use an airport’s runway(s) for landing or takeoff (i.e. demand) than the airport can handle (i.e. capacity), queuing (read: delays) results. For a better explanation, you can check out our posts on airport capacities, queuing in the airspace system and how delays are distributed. Like the former outlooks, we remain focused on identifying imbalances between arrival capacity and demand, though arrival delays typically flow through to departures3 at that airport.
This is different from the MVP we mentioned in Where we’re going: that’s underpinned by some pretty sophisticated machine learning from Yamaç and will be productized outside of Substack. This iteration is Excel- and Tim-based, which carries some limitations (namely, size of the dataset). For that reason, we’ve narrowed our focus to the ten most EDCT-prone hubs4. And because it relies on a filter-like mechanism more than inference, when estimating how frequently an arrival demand overage will occur in a given hour, we can sometimes end up with pretty small samples of similar past capacities. To address this, we’ve applied weights such that hourly estimates with small underlying samples contribute less to conditional daily probabilities (i.e. what is that chance that a demand overage will occur in at least one hour of the day). We’ll tune these weights5 once we’ve accumulated enough forecasts to perform verification.
A couple other technical notes:
Historical hourly capacities reflect the period October 31, 2021 to August 8, 2022. We’ll append more recent data every month or so. Capacities from non-reportable (i.e. overnight) hours are excluded, though we don’t control for runway closures.
We’re using a modified version of efficiency AAR to measure historical arrival capacities. In cases where the ASPM’s arrival demand metric is less than efficiency AAR, then we take efficiency AAR as is. In cases where the arrival demand metric is greater than or equal to efficiency AAR, we take the lesser of efficiency AAR and landed; this is done so that capacity is adjusted downwards when fewer aircraft are landed than the advertised rate.
We don’t consider the valid time for a forecast hour nor demand when filtering for similar past capacities, so we don’t capture an airport’s ability to “flex-up” capacity to meet demand. This will be particularly impactful for Newark (EWR), where modal capacity is 40, though scheduled demand typically exceeds 40 once or twice per day; the airport can (briefly) run at a 48-rate to accommodate these peaks.
The NBM provides probabilities of snow and thunder, though observed weather is binary in that respect (i.g. snow/thunder was either present or not). We’ve elected to match on observations with snow or thunder present if the forecasted probability is at least 10%, though this may produce unreasonably bearish estimates for hours when there’s a low-end (e.g. 10-30%) chance for snow or thunder.
We’ve attempted to distill the most important information about risk (where risk is a function of likelihood and consequence) into a table for readers to glance at; we’ll sandwich it between weather graphics. The first two columns are intended to communicate information about the chance of an arrival demand overage (and therefore delays):
Probability that an overage occurs in at least one hour reflects the cumulative likelihood across the entire day. In a binary sense, it answers the question is disruption likely at that airport on that day?
Count of hours where an overage is more likely than not answers the question, is any capacity/demand imbalance widespread or more isolated?
If the first two columns are meant to communicate how likely, then maximum overage, given capacity in the 10th percentile is meant to communicate how bad. More specifically, it considers scheduled arrival demand as a percentage of a reasonable worst-case capacity. A relatively short overage (10% or less) might be resolvable with a largely imperceptible form of traffic management (e.g. metering) or perhaps a brief, first-tier ground stop; a taller overage likely requires a weightier initiative (e.g. ground delay program). A negative number indicates that demand falls short of even 10th percentile capacity.
We’ll do our best to highlight any travel waivers that airlines have issued (we might share these via Twitter if the airline publishes the waiver after we publish a post). Otherwise, we’ll take the opportunity to remind readers that airlines have meaningfully improved general rebooking flexibility by eliminating change fees for most tickets (though the fare difference may still apply). We’ve linked to the same-day change policy for American, Delta, United and Southwest and JetBlue.
Alright—enough background and methodology. Let’s get to the new format. (And again, feedback is encouraged!)
Wednesday, August 10
We forecast TSA will screen 2.181 million travelers (± 0.5σ, or a prediction interval of about 38%, is 2.12-2.24 million travelers).
A cold front approaches the NYC area today, slowly moves across tonight, then stalls over the Mid-Atlantic on Wednesday. Depending how far south the front sinks, a few showers are possible during the day. By evening, a weak wave of low pressure develops along the stalled front: ample moisture remains in place, though greatest risk for torrential rainfall looks to be south and west (i.e. the DC area). Speaking of Washington, the cold front will not begin to track across the area until early Wednesday. Cloud cover and shower coverage is forecast to increase through the morning hours before convection develops by late afternoon.
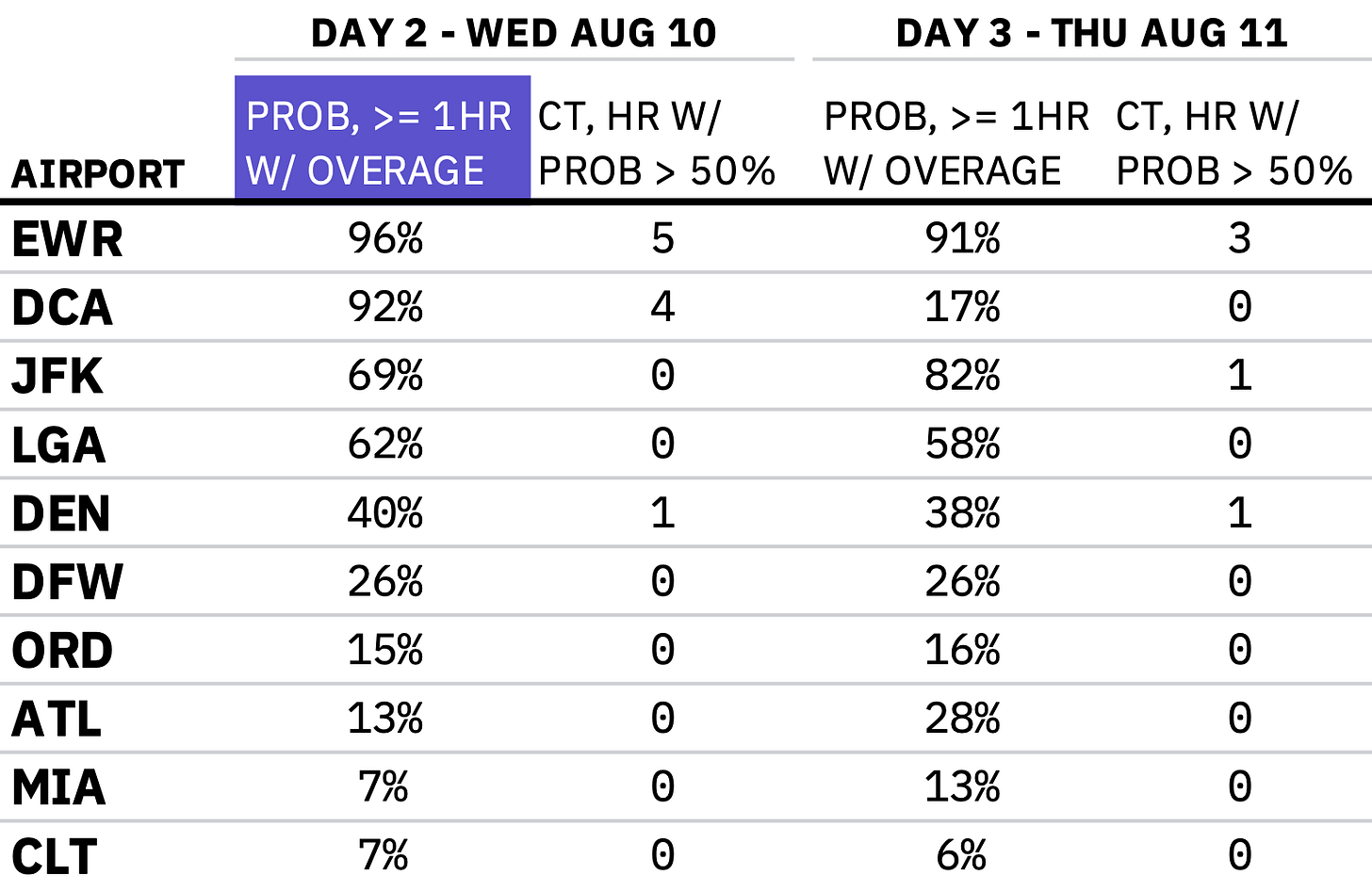
Elsewhere, scattered monsoonal showers and thunderstorms are expected across the Southwest (we’ll be watching LAS, even though it’s not part of our automation).
Thursday, August 11
We forecast TSA will screen 2.395 million travelers (± 0.5σ, or a prediction interval of about 38%, is 2.33-2.46 million travelers).
On Thursday, conditions in the WAS-NYC corridor begin to dry out, though a steady northwest flow may pressure airport capacity (especially at EWR). Out West, the pattern remains largely unchanged: for DEN specifically, the Boulder Weather Service Office couldn’t rule out an afternoon or early evening thunderstorm owing to meandering high pressure.
You can check out hourly estimates in this workbook.
TSA has screened an average of 1.72 million travelers on Tuesdays, trailing Saturdays (second to last in TSA throughput) by 4%.
Airlines respond by scheduling fewer flights, which reduces demand on the NAS. This reduced demand flows through a lower air traffic delay incidence: 1.48% of Tuesday arrivals to core 30 were assigned an EDCT (see footnote 4), lowest by 32 basis points (again, Saturdays were next closest).
Cargo airlines as well as private jets are not included in scheduled demand and only become apparent when they file a flight plan (generally day-of). This unforeseen demand introduces the risk that delay probabilities/intensities are under-forecast.
Consider a scheduled "turn" at an airport: the inbound flight is scheduled to arrive at 2:19 p.m. and departs at 3:30 p.m. (71 minutes of turnaround time). Let's say the inbound is delayed by 40 minutes and instead arrives at 2:59 p.m. We'll further assume that the airline doesn't need the full 71 scheduled minutes to turn the aircraft and can accomplish the turn in 45 minutes if they hustle—the departure will push back from the gate at 3:44 p.m. (delayed by 14 minutes). In this example, a 40 minute arrival delay in the 2 p.m. hour is partially passed through to a departure in the 3 p.m. hour. Had the turnaround been scheduled at 45 minutes instead (i.e. no turnaround buffer), the lag between arrival and departure delay would still exist, however the delay would be fully passed through.
Additionally, our efforts are aimed at diagnosing air traffic delays (i.e. those that result from an imbalance between capacity and demand). Though not the focus of our efforts (yet), delays owing to aircraft servicing, airline staffing, network effects, etc. are always lurking.
From October 31, 2021 to August 5, 2022. We used the percentage of arrivals to Core 30 airports assigned an estimated departure clearance time (EDCT) as our measure of air traffic delay incidence. EDCTs (often called wheels up times) marshal the queueing that results when demand for an airport’s runway(s) exceed their capacity.
You could quibble with our definition of hub: we skipped BOS, FLL, LAS, MCO and TPA, which all have a higher EDCT incidence than ATL. We also elected to exclude PHL and SFO for now—though they were (are?) true connecting hubs, their laggards in flight schedule recovery.
For starters, we’re using the cubed root of that hour’s sample size as a percentage of the cubed root of that airport’s largest hourly sample size
Regarding feedback: I'm all for increased frequency. As you know, Airline Operations is what I do for a a living, and this is the only place I get data-heavy info like this
Tim,
I think you may need to broaden your aperature on delay and methods of traffic management? Delay in-and-of itself is not necessarily the root of inefficiency.
There are many sub-metrics to GDP use that, (while not in the public domain), are useful in determining the planning and execution aspects of a GDP.
A short list includes:
- Was the GDP implimented within 2 hours of "start-time"
- Did a Ground Stop precede the GDP
- Did the FAA use a variable AAR, or was the assumption that all hours have equal capacity
- Was the GDP rate modified after implimentation
- Was the GDP cancelled early
Delay should be used as a tool to an acceptable outcome when capacity is constrained. GDPs can be effective when used properly. However, they have to be viewed in the context of other impacts such as completion rate, airborne holding, ground stops, diversions, etc, etc.
Just my opinion.....